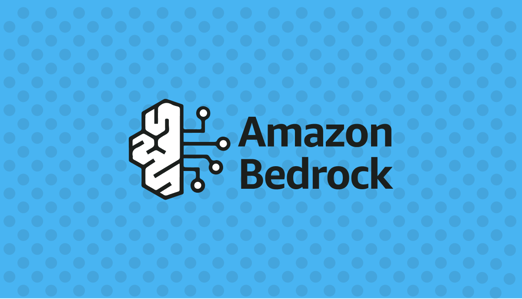
AWS structures its AI/ML offerings into three layers: infrastructure, generative AI platforms, and applications. At the foundation is the infrastructure tier, featuring hardware and Amazon SageMaker for building and deploying ML models. The middle layer, Amazon Bedrock, offers access to leading large language models (LLMs) and other foundational models, enabling businesses to scale generative AI applications efficiently. The top layer includes user-friendly applications that leverage generative AI without requiring specialized knowledge or coding.
Other vendors like Google Cloud Platform (GCP) and Microsoft Azure also offer generative AI solutions, each with their own unique capabilities and advantages. However, AWS Bedrock is gaining prominence due to its comprehensive features and seamless integration with other AWS services.
As AWS Bedrock gains importance, understanding its basics and pricing model becomes crucial. This allows businesses to effectively utilize Bedrock capabilities while managing costs, ensuring they maximize the benefits of AWS's powerful AI tools. This blog will explore the basics of AWS Bedrock, its fundamentals, pricing model, and challenges for Bedrock cost optimization.
This is one of our most popular articles. Don’t miss another highly viewed piece on What is Datadog—read it here.
What is AWS Bedrock?
AWS Bedrock is a fully managed service designed to simplify the development of generative AI applications. It provides access to a selection of high-performing foundation models (FMs) from top AI companies, all accessible through a single API. These foundation models are adaptable AI models trained on large datasets to perform various tasks without requiring retraining for each new task. They excel at comprehending natural language inputs and generating text or images as outputs but need direction for complex tasks.
Bedrock offers a comprehensive set of tools and capabilities essential for building secure, private, and responsible AI applications. Developers can experiment with different FMs, customize them using their data through techniques such as fine-tuning and Retrieval Augmented Generation (RAG), and create agents that interact with enterprise systems and data sources.
Additionally, its serverless nature eliminates the need for infrastructure management, ensuring seamless integration with existing AWS services. This reduces operational overhead and allows businesses to focus on developing AI applications. Bedrock automatically scales to handle varying workloads and integrates smoothly with services like Amazon S3, AWS Lambda, and Amazon SageMaker.
Image Source: Amazon Bedrock
Risks of Adopting AWS Bedrock Without Understanding Its Cost Structure
Using AWS Bedrock without a thorough understanding of its cost structure can lead to bill shockers. The complexity of its pricing models, such as on-demand and provisioned throughput, can be confusing. Without clear insight into usage patterns and cost drivers, it's hard to predict costs accurately, leading to budget overruns and reduced ROI.
Additionally, the varying nature of AI workloads can cause fluctuating costs, making it difficult to leverage cost-saving opportunities. Thus, to avoid financial surprises and get the most out of AWS Bedrock, it's important to fully understand its pricing and have strong cost management strategies in place.
AWS Bedrock Pricing Models
AWS Bedrock offers various pricing options to fit different needs and budgets. Understanding these options can help you manage costs effectively. Here’s a basic overview:
- On-Demand Pricing
With on-demand pricing, you pay only for what you use without any long-term commitment. This model charges based on the number of input and output tokens processed. This flexible pricing suits users with changing workloads. For more details on model-based pricing, you can check the AWS Bedrock pricing page. - Provisioned Throughput Pricing
For users with more predictable and consistent usage patterns, the provisioned throughput pricing model offers a way to reserve capacity and ensure guaranteed throughput. This model is billed by the hour per model unit, with options for 1-month or 6-month commitments. This option provides cost savings for steady, long-term projects in exchange for long-term commitments.
-
Batch Processing
Batch mode allows you to process large-scale processes by submitting a set of prompts as a single input file. The responses are stored in an Amazon S3 bucket for later access. Pricing for batch processing is the same as on-demand pricing, making it suitable for handling simultaneous large-scale predictions efficiently.
-
Model Customization
Amazon Bedrock enables you to customize foundation models (FMs) with your data to deliver tailored responses. Customization can be done through fine-tuning with labeled data or continued pretraining with unlabeled data. Costs include charges for model training based on the total number of tokens processed and monthly storage fees for custom models. Inferences using customized models require the purchase of provisioned throughput, with charges based on the number of hours used.
- Model Evaluation
Model evaluation on Amazon Bedrock is billed based on actual usage without volume commitments. Automatic evaluation incurs charges only for the inference from the chosen model, with algorithmic scores provided at no extra cost. For human-based evaluations, charges include model inference costs and a fee per completed human task. Evaluations managed by AWS are customized, with pricing determined through a private engagement with the AWS expert evaluations team.
The Challenges of Forecasting and Managing AWS Bedrock Costs
Effectively forecasting and managing AWS Bedrock costs involves addressing several key challenges. These stem from the complexity of machine learning (ML) workloads and the dynamic nature of generative AI applications. Key challenges include:
1. Lack of Visibility
One of the primary challenges is the lack of granular visibility into spending. Without detailed insights into where, how, when, and by whom resources are being consumed, it becomes difficult to create accurate forecasts and manage cloud costs effectively. This lack of transparency can lead to inefficient cost management strategies, as organizations struggle to pinpoint specific areas for optimization.
2. Dynamic Usage Patterns
Generative AI applications often experience fluctuating usage patterns, influenced by factors such as user demand and the specific tasks being performed. Additionally, usage patterns can be affected by uncontrollable factors like organizational policy changes, cost constraints, or modernization. These unpredictable changes make it challenging to forecast costs accurately. Without efficient forecasting, organizations may struggle to leverage discounting opportunities with predictable usage patterns, thereby missing out on potential cloud cost savings.
3. Complexity of Cost Structures
AWS Bedrock's cost structure includes multiple components: data storage, compute resources, data processing, model training, and inference. Managing these cost drivers requires careful attention. Simplifying the approach to managing these components can be challenging, particularly for businesses new to ML operations.
4. AWS Bedrock Cost Management
Effectively managing AWS Bedrock costs involves continuous monitoring, analysis, and optimization of resource usage. This process can be tough, as businesses need to stay vigilant in tracking their usage, identifying inefficiencies, and making necessary adjustments to optimize for cost efficiency every hour. Failure to do so can result in unexpected cost overruns and reduced overall efficiency of AI/ML operations.
Addressing these challenges requires a strategic approach to cost management. This includes using tools for detailed forecasting and actively optimizing resource usage.
How Finout Can Help Manage and Optimize AWS Bedrock Costs
Managing and optimizing AWS Bedrock costs can be challenging, but Finout offers a solution to simplify this process. With real-time cost monitoring, automated cost allocation, and usage analytics, Finout provides comprehensive visibility into your cloud spending. Customizable dashboards and alerts help you stay on top of expenses, identify inefficiencies, and take immediate action. These features empower you to manage AWS Bedrock costs effectively, optimize your budget, and maximize the value of your cloud investment.
When you integrate AWS Bedrock with Finout, your costs will be seamlessly tracked and displayed within the Finout dashboard. For more information on how Finout manages these costs, visit our Artificial Intelligence page.
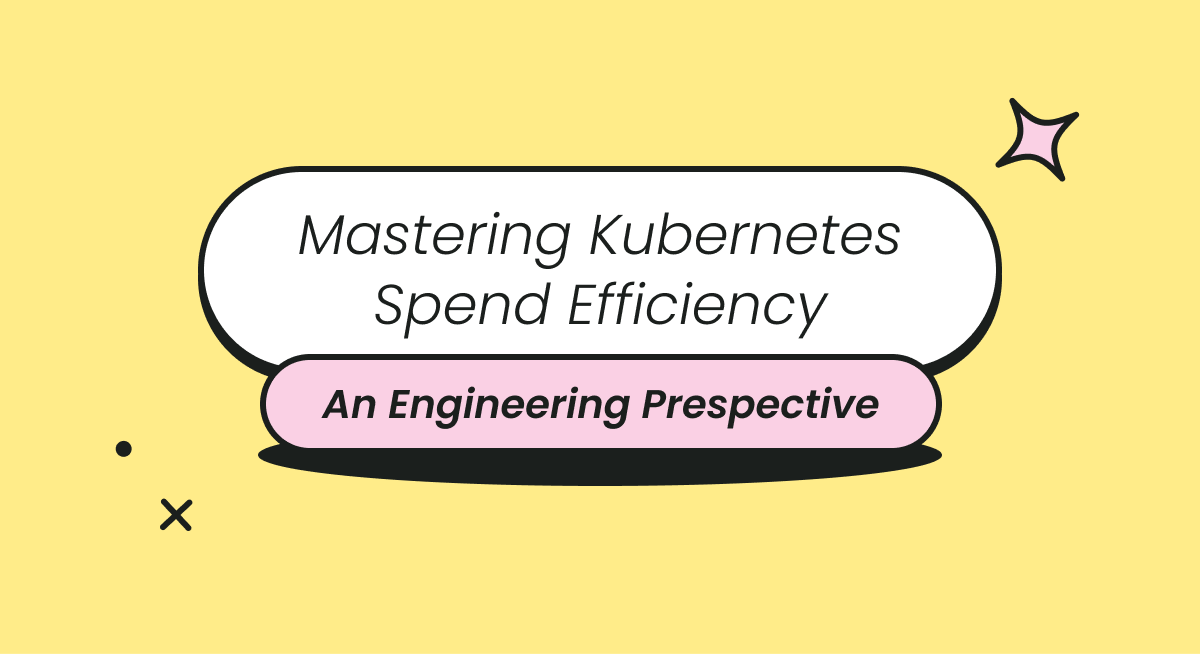
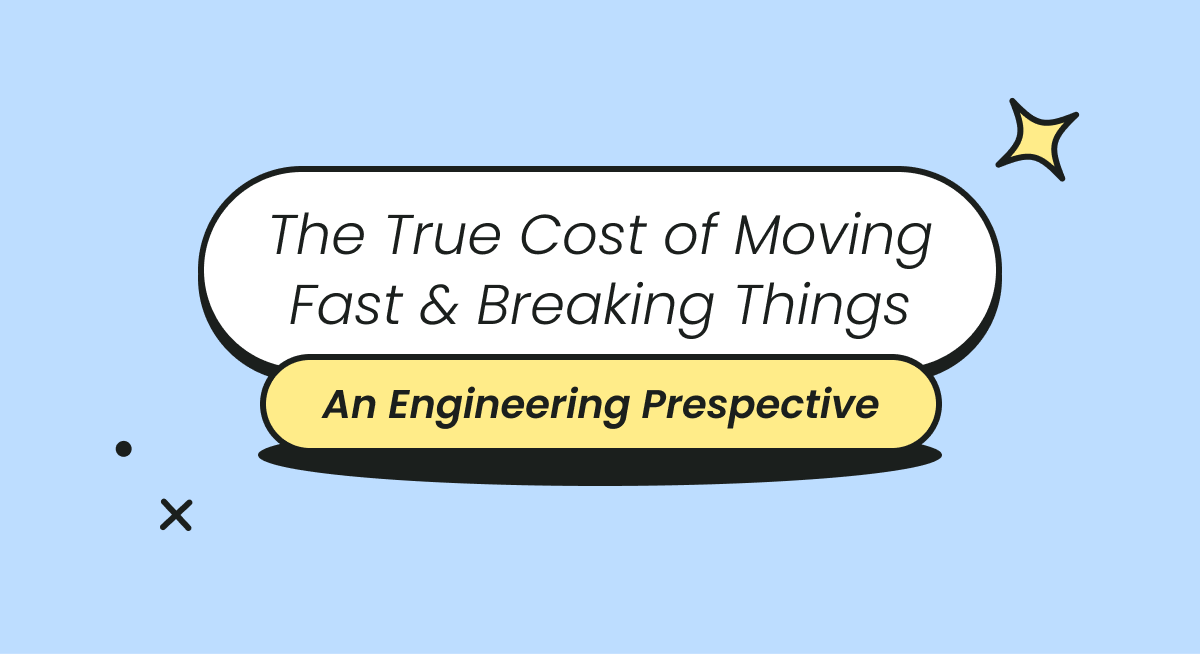
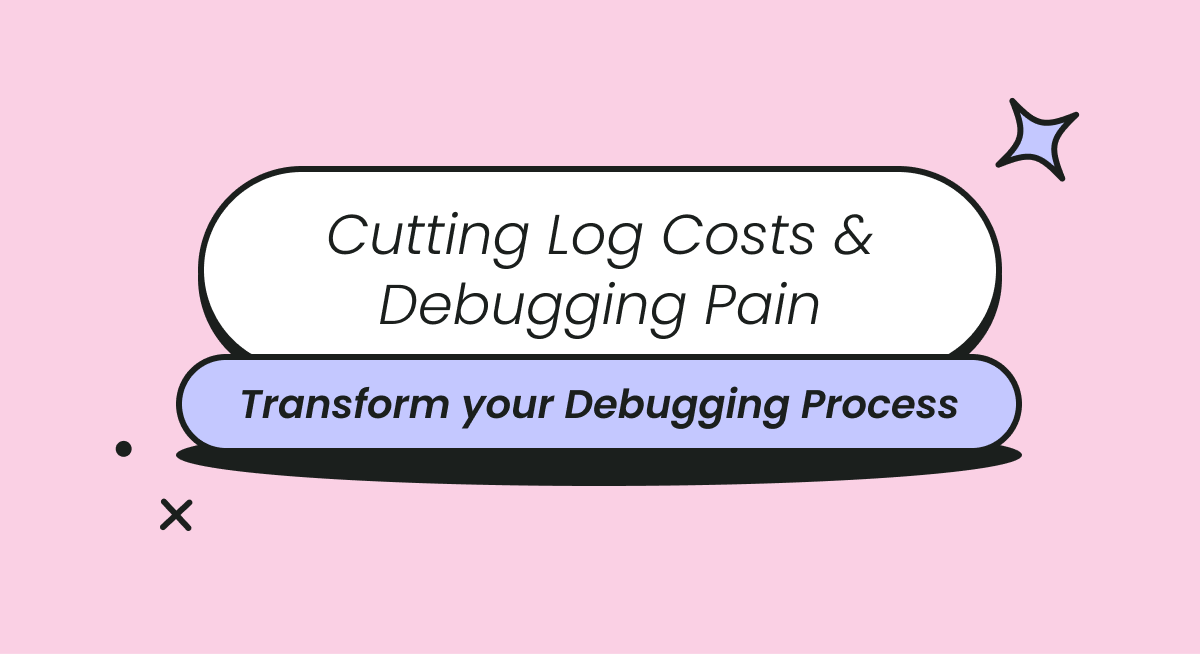
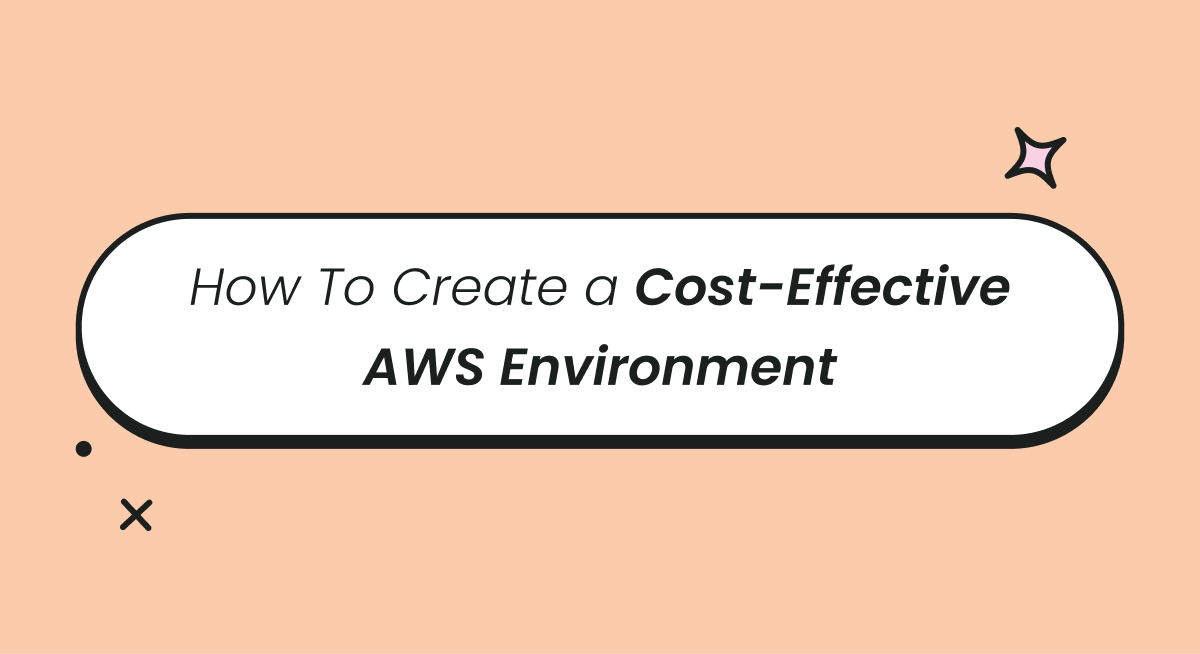
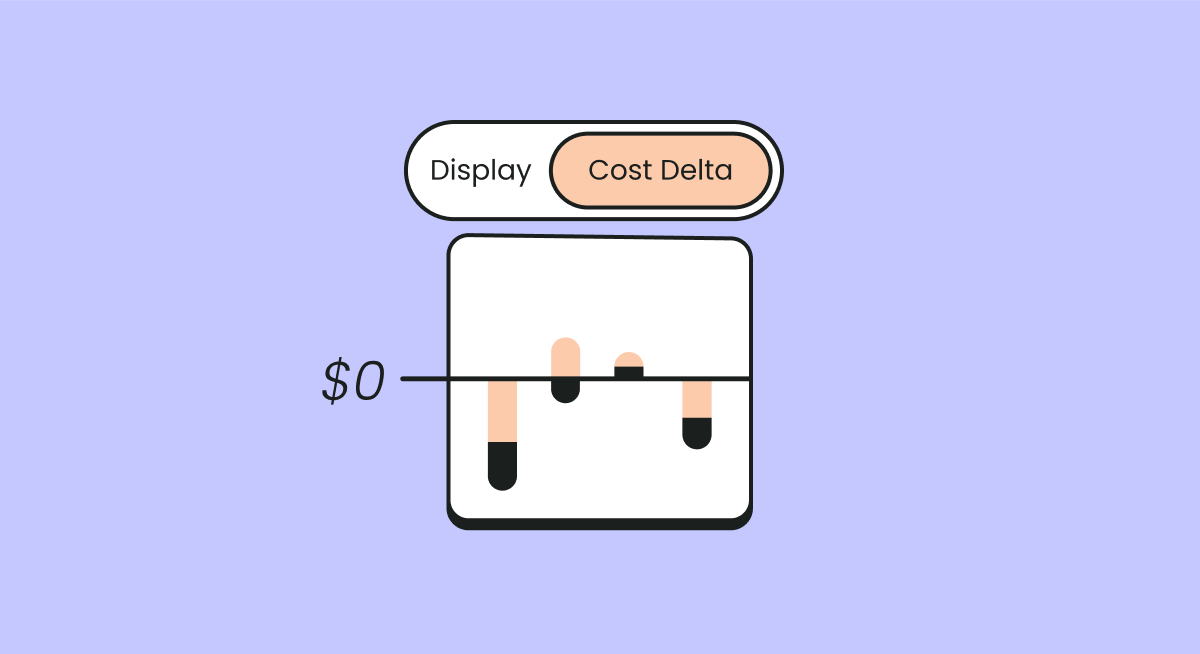
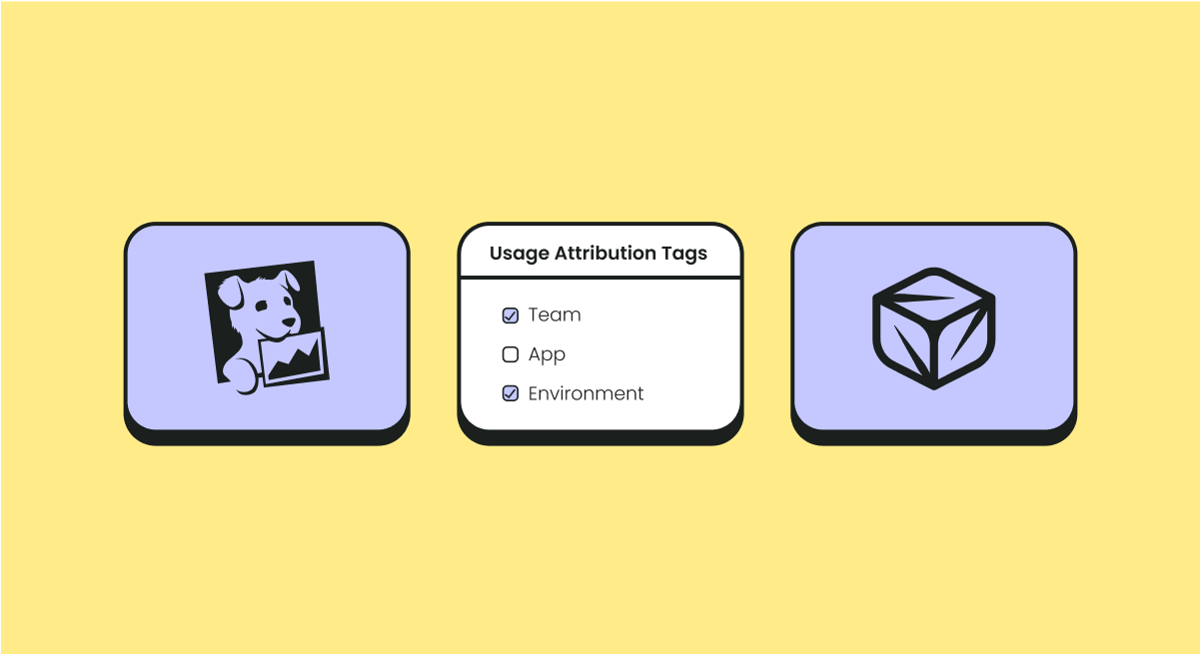